As a business owner, you probably already know that out-of-home advertising (OOH) is one of…
The measurement of how a marketing campaign affects a critical indicator is called lift analysis. Engagement, in-app spending, and conversion frequency may all be tracked in mobile marketing. All of these are essential for successful mobile billboard advertising. Curious about how to calculate marketing lift? To compute lift, you divide the percentage change in each statistic by the percentage change in the control group.
If you want to know which group is better off, you compare the group that received the marketing campaign (the lift group) to the group that didn’t get the campaign (the control group). A control group is a subset of your users who don’t receive special communications for benchmarking purposes.
NOTE: There are a few considerations to consider while doing an actual a/b test, such as one where the treatment group and the control group are equal. As long as you’re mindful of data problems like limited sample sizes that could skew your conclusions, this form of marketing lift research is still valuable.
How is Lift Analysis Used
In-app notifications and push notifications both have the potential to be robust growth engines. However, not all methods are equal.
Some campaigns have been demonstrated to affect user engagement and conversions negatively. A lift analysis can be used to aid in the termination of unsuccessful campaigns and improving successful ones. If you’re calculating lift marketing, you need a control group to ensure that you’re getting an accurate reading.
1. Using Lift Analysis For a Short-Term Effect
Consider a scenario in which you have an e-commerce app and execute a campaign to give a 20% discount to consumers through push notification. You A/B test the 20% off offer with two different alerts since you’re a marketer who experiments. The control group does not get the 20% off promo.
Message A: All of our comfortable winter sweaters are 20% off with the coupon SHOP20 during this flash sale. Find the ideal gift or outfit. Get your shopping on while supplies last.
Message B: With the code MERRY20, you can get 20% off all of our most popular accessories today only. Hurry up, or you’ll miss out on all the fun! Give yourself a break.
In this example, users who do not get promotional notifications convert at a 4% rate. Your discount campaign may now be measured against this baseline data.
A 10% conversion rate from Message A allows you to compute an increase of 150% compared to the control group after the campaign has run. Message A seems to have a good effect on the number of people that sign up.
However, Message B’s conversion rate is just 3.5%, which translates to 14% fewer engagements than the control group. To put it another way, message B affects the conversions negatively.
In this scenario, intelligent marketers would stick with Message A due to this response rate and stop using Message B.
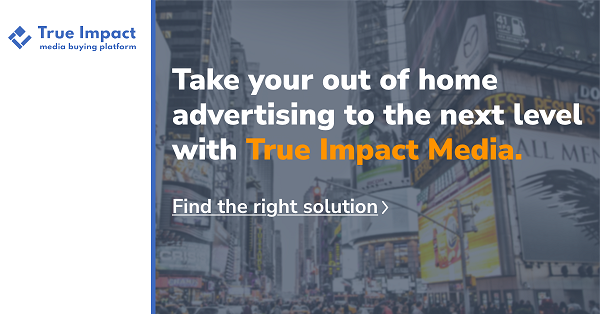
2. Using Lift Analysis For a Long-Term Effect
An in-app messaging campaign to offer your premium version for seven days at no cost may be used as part of your media app business model. Control groups that do not get the free trial offer are already in place. You also run two A/B tests for the new campaign’s in-app messaging since you believe in testing everything.
Message A: Offline listening is available for those who want it. Skip the commercials. In addition, you’ll be able to listen to any music you choose. You can check out our premium edition for FREE for a week by clicking here. You don’t even need to have a credit card to get started. Try it now.
Message B: Our premium edition is now available for a free 7-day trial! Get the most out of your music experience by clicking here and taking your music everywhere. There aren’t any restrictions, so enjoy as many songs as you like.
“Listening to music” is a significant conversion event in this case. Message B users listened to more songs the following day than those who got Message A in the early findings (150% lift over control). There is an initial increase, but it diminishes quickly and returns to its normal level.
Those who got Message A could listen to more songs throughout the free trial period than those who received Message B, even when looking at the long-term consequences of each campaign.
An intelligent marketer would keep running Message A while discontinuing the in-app message campaign using Message B.
Don’t get caught up with how well your communications are received right away. Over time, see which campaign has the most impact on the Key metrics that matter most to you. If your efforts are making an impact, then lift analysis is a great way to find out. It’s impossible to view the whole picture without lift analysis.
Validation of How Lift Analysis Improves Messaging Campaigns
Data is always reliable, and it does not lie.
The effectiveness of lift analysis has been studied, and results reveal that in the first month, businesses witnessed an average 32% increase in engagement (sessions per user) and a 20% increase in conversions when employing lift analysis.
Although these data show that push and in-app messages are effective avenues for promoting app growth, not all techniques are the same. The investigation also reveals that specific campaigns had a negative effect on the levels of participation and the number of sales.
Consequently, lift analysis is so critical to the success of marketers since it allows them to rapidly identify and delete initiatives that aren’t performing and keep improving the ones that are. The only method to accurately measure the effect of a project is to use a control group.
Reasons Why Lift Charts Are Valuable
A slew of indicators may be used to evaluate the effectiveness of machine learning models. It’s essential to know things like precision-recall (ROC curve) and accuracy. Some are helpful, while others may mislead or fail to address the issue.
A reasonable statistic for balanced classes would be accuracy, where each label has approximately the same amount of instances; however, this is entirely deceptive for unbalanced classes. However, data scientists must constantly deal with varying types, such as forecasting whether or not someone would purchase anything in an online store. Even if just two consumers out of every hundred make a purchase, the model can easily make the prediction that every client will not make a purchase and yet obtain an accuracy of 98%! The model’s quality cannot be judged in this manner.
However, additional measures like accuracy and recall might also help you understand your model. Lift analysis, a valuable tool for evaluating performance, is also worth exploring further. Using a basic churn model, we’ll see whether a consumer of an online platform will decide to quit its membership or not. To solve this issue, the user voluntarily cancels their subscription (churn=1) or retains it (churn=0).
The underlying premise is that data should be grouped depending on the projected chance of churn (value between 0.0 and 1.0). There are typically ten groups: 0.0 to 0.1, 0.1 to 0.2, 0.3 to 0.4… 0.9 to 1.0.
The actual turnover rate for each category should be calculated. In other words, you divide the total number of clients in each group by the number of persons who left each group.
The model’s goal is to predict how probable a customer would terminate its service. As a result, our projected (churn) probability should be proportional to the actual (churn) probability so that a high predicted score should be associated with a high real (churn) rate. The opposite is true if you want to know that a client won’t churn based on a model’s prediction.
A surge in the lower-scoring groups would tell you that your model is flawed and does not accurately represent reality. If this were the case, the churn rate would drop exclusively as the score decreased. Lift analysis, of course, can only go so far. If you can’t figure out what’s causing this issue, you’ll have to solve it yourself. The only thing left is to return to the lift chart and see whether the quality has improved since the model was last updated.
Key Takeaways
One of the most important things you can learn from this is to look beyond the immediate results of your mobile messaging efforts. Instead, keep track of which campaigns have the most significant overall improvement in key performance indicators. With lift analysis, you can measure the actual effect of push notification and in-app message campaigns and see how effective they are.
Sources:
- Data Science. https://www.researchgate.net/publication/342678200_Data_Science
- Engaging the unengaged customer: The value of a retailer mobile app. https://www.sciencedirect.com/science/article/pii/S0167811619300242
- The Effect of Timing and Frequency of Push Notifications on Usage of a Smartphone-Based Stress Management Intervention: An Exploratory Trial. https://journals.plos.org/plosone/article?id=10.1371/journal.pone.0169162
- Mobile marketing campaigns: practices, challenges and opportunities. https://www.inderscienceonline.com/doi/abs/10.1504/IJBIR.2020.105996
- Engagement with Social Media and Social Media Advertising: The Differentiating Role of Platform Type. https://www.tandfonline.com/doi/full/10.1080/00913367.2017.1405754
- Marketing research on Mobile apps: past, present and future. https://link.springer.com/article/10.1007/s11747-021-00815-w